Smart Holiday Shopping with Amazon’s Recommendation Engine
At Christmas time many of us are struggling with choosing the most suitable gift for our loved ones. Gift hunting for Christmas may require days of planning, sometimes weeks. Apart from its certain fun and joyful part, Christmas shopping can be a time-consuming and stressful experience.
Luckily in the age of advanced technology, there are freely available tools that can make the shopping process much more efficient and productive. In this post I will show you how one of the world’s largest retailer site, Amazon.com can assist you in finding the best gifts for your friends and family within a reasonable timeframe with the help of its smart recommendation engine.
Personalized User Experience
The world’s most successful websites such as Amazon, Facebook, and Youtube are so popular because they offer a personalized user experience to everyone.
Personalizing user experience basically means that companies observe their users while they navigate through their site and perform different actions on it. They collect the data into neat databases, and analyze it.
Isn’t that harmful for privacy? From a certain point of view, yes it is; these companies may know more about us than our closest friends or even ourselves. On the other hand, they offer us a service that can make our lives easier, and our decisions better-informed.
If we look at it from a transactional point of view we "pay" for an enhanced user experience and comfort, with a part of our privacy.
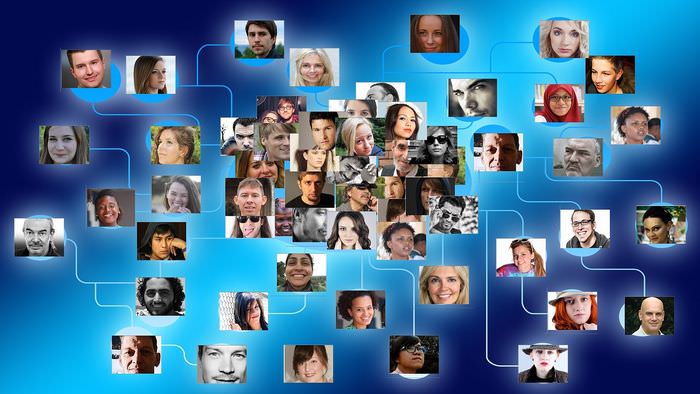
Of course, legal battles between online content providers and authorities are constant, just think about the not so loved EU cookie law, but as opting out is less and less a realistic option for someone who wants to enjoy a 21st century lifestyle, it can be useful to understand how personalized recommendations work behind the scenes.
The Tech Behind Amazon’s Recommendations
While navigating through Amazon’s website, we can find personalized recommendations everywhere under headings like “New For You”, “Recommendations For You in Kindle Store”, “Featured Recommendations”, “Customers Who Bought This Item Also Bought”, and many others.
Recommendations have been integrated into every single part of the purchasing process from the product lookup to the checkout. Customized recommendations are powered by an intelligent recommendation engine that gets to know users better and better as they use the site.
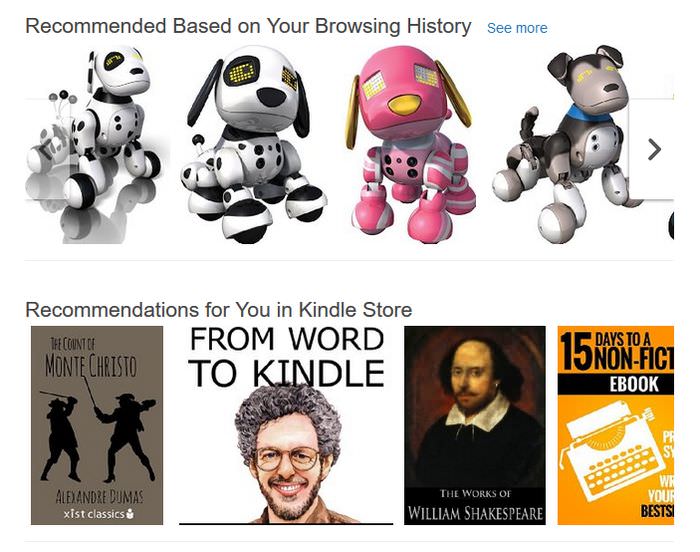
To understand recommendation systems better, it’s a good idea to think about them as advanced versions of search engines. When we look up an item on Amazon, it doesn’t only return the results, but also makes predictions about the products we may need, and shows its recommendations for us.
Recommender systems use different kinds of machine learning algorithms, and they have become commercially implementable with the evolution of big data technology. Recommendation engines are data-driven products, as they need to find the most relevant small dataset in the huge ocean of big data.
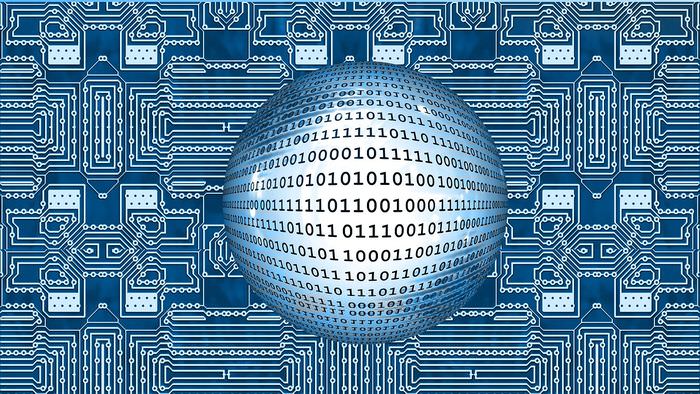
The computational task that recommendation systems need to solve is the combination of predictive analysis and filtering
They use one of the following approaches:
(1) Collaborative Filtering, that looks for similarities between collaborative data such as purchases, ratings, likes, upvotes, downvotes in:
- either the user-user matrix, where recommendations are generated based on the choices of other customers who liked, purchased, rated, etc. similar products,
- or the product-product matrix, where the recommendation engine returns products that are similar in purchases, likes, ratings, etc. to the products the current user has purchased, rated, liked, upvoted before
Amazon uses the latter, as it’s more advanced (see in detail in the next section).
(2) Content-Based Filtering, that makes predictions based on the similarities of objective characteristics of products such as specifics, descriptions, authors, and also on the previous preferences of the user (that here are not compared to the preferences of other users).
(3) Hybrid Filtering, that uses some kind of combination of collaborative and content-based filtering.
The Product-Product Matrix
The traditional way of collaborative filtering makes use of the user-user matrix, and above a certain amount of data it has serious performance problems.
To match the preferences, ratings, purchases of all users, and find those who are the closest to the active user, the recommendation engine has to analyze every user in the database and match them against the current one.
If we think about Amazon’s size, it’s clear that this kind of filtering isn’t feasible for them, so Amazon’s engineers developed an upgraded version of the former method, and called it item-to-item collaborative filtering.
Item-to-item collaborative filtering keeps collaborative success as the benchmark instead of the objective qualities of a product (see content-based filtering above), but runs the queries in the product-product matrix that means it doesn’t compare users, instead it compares products.
The recommendation engine takes a look at the products we have purchased, rated, put onto our wish list, commented on, etc. so far, then looks up other items in the database that has similar rates and purchases, aggregates them, then returns the best matches as recommendations.
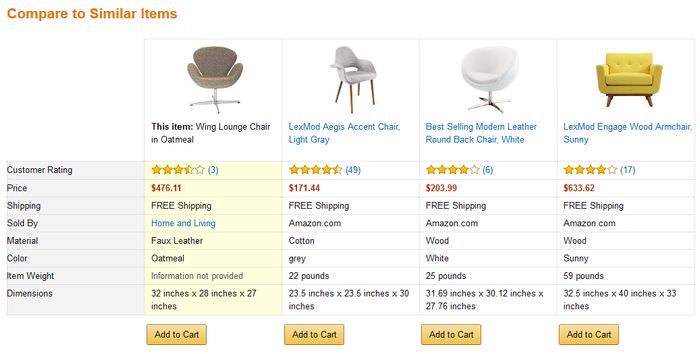
How To Get Better Recommendations
Back to Christmas shopping, it’s possible to train Amazon’s recommendation engine to get better results. If you only have a vague idea about what to buy for a loved one, you don’t have to do anything else other than leaving traces on the website while browsing.
For the sake of this post I’ve tried this out by myself.
My starting point was that I wanted to find some smaller office furniture, but didn’t exactly know what. So I entered some related keywords into the search bar, and began to browse the results. I put the items I liked into my wishlist, rated some reviews as “Helpful”, dropped some office furniture into my basket.
If I’ve ever bought a similar item on Amazon, it would have been quite useful to write a review on it, but actually I couldn’t do that (you can only write reviews on products that you’ve already purchased).
After about 10-15 minutes I stopped, and clicked on my Recommendation pages (which can be found under the “[Your Name]’s Amazon” menu point). Before the experiment I only had books on this page, as that’s what I usually buy on Amazon. After my extensive lookup, the books have disappeared and have been replaced by cool office furniture, as you can see below.
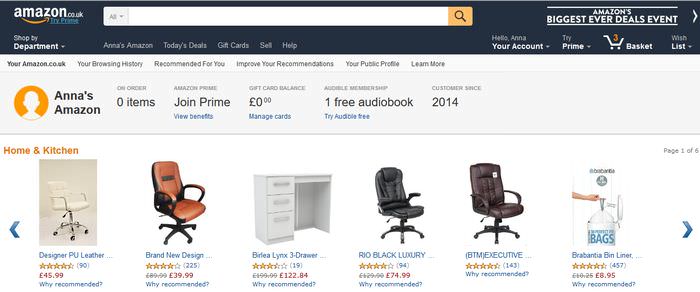
Tweaking The Engine
It’s possible to further train the recommendation engine, as below each recommendation there’s a “Why recommended?” link. Among my recommendations you can see a bin liner (last item), which isn’t an office furniture product and one I don’t want to buy for Christmas.
So let’s see why it’s here.
After clicking on the link, Amazon informs me it was recommended because I put a certain office computer chair into my basket. Well, that’s an interesting connection, but I still don’t need it.
I have two options here, I can either tick the “Not interested” checkbox next to the bin liner, or the “Don’t use for recommendations” next to the office chair. I tick the “Not interested” checkbox.
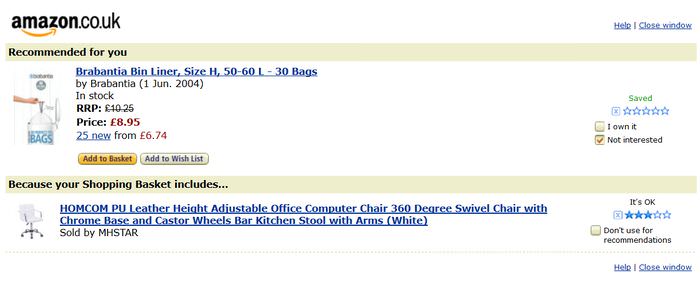
And at this point the bin liner has disappeared, replaced with another recommended product, which means I’m one step closer to the perfect gift.
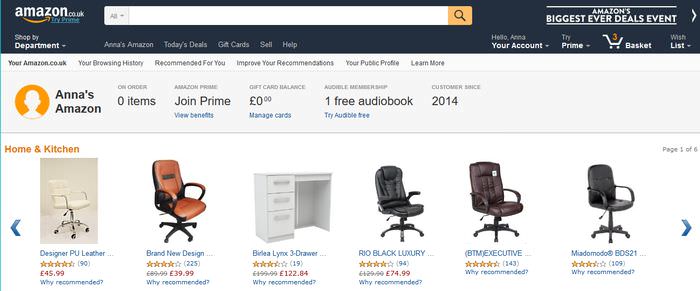
Too bad if I’ll ever need that exact bin liner in the future though. Oh wait. I’ve found the solution for that. Under the “Improve Your Recommendations” menu point, I can edit the items I’ve marked with the “Not interested” label
When I have found my imagined gift hunt, I can simply uncheck the products I may want to see among my recommendations in the future.